The Ancients used to say that “knowing thyself” was the key to success in life; in marketing, there could be a similar saying: “Know thy audience.” This might seem like a banal motto, but much like the earlier philosophical phrase, it hides behind it a surprising amount of depth.
After all, when you have a mass-market product, you could feasibly try to sell it to the entire world. But this approach is untargeted and likely to waste a lot of time and money. As such, you need to understand who the audiences are that would be interested in your product.
In this article, I will explain the value of using In-Market audiences. This is especially beneficial if you have a mass-market product and want to deliver media buying through YouTube. I will also explain how to use Custom Intent/Custom Affinity if you have a more niche product.
Think of this not so much as a “How To Do X” Guide but a manual on maximizing the potential of your campaigns via targeting and insight tools developed by Google.
INDEX
Why YouTube Advertising is (Wrongfully) Overlooked
The Different Ways Google Targets Audiences
Why YouTube Advertising is (Wrongfully) Overlooked
Revenue growth is the thing that is stressed the most in marketing circles, and a lot of time is spent trying to maximize this metric. But it’s easier said than done in this era of hyper-segmentation when there are potentially millions of groups to target and each of these will have different behaviours.
As such, achieving revenue growth becomes a complicated affair, and there are many ways of doing it. The most typical method is to simply improve the quality of the ad copy and be more convincing than competitor messaging.
But this method has its limits. Past a certain threshold, there are diminishing returns for optimizing the ad copy because no matter how convincing you are, only a certain portion of your existing audience would ever buy your product. You’re preaching to the choir, as it were.
As such, while remarketing is an incredibly powerful tool, it has its limitations.
The other option is to expand your potential audiences by increasing the number of places where your prospective clients can interact with your brand. As an avenue for growth, this is often given secondary consideration. Many marketers assume they’ve exhausted all marketing avenues.
This is far from the case!
YouTube, for example, remains a relatively unexplored territory, as it is sometimes willfully overlooked. There have been several reasons for this, but to summarize a complex issue, it’s just been erroneously perceived as a minefield.
Initially, advertisers believed that YouTube could not deliver direct results and conversions at all. They failed to understand that, much like with other advertising, the message is just as important as how it’s delivered. You can’t just port the same television advertisements that you were previously using, completely change how they’re experienced, and expect the same results.
Imagine seeing 2001: a Space Odyssey (or any film that depends on grand visuals) on your phone while riding the subway on your commute to work – instead of the grand movie screen with an orchestra blaring at such volumes that your internal organs vibrate. In no shape or form would it be nearly as impactful as it might have otherwise been originally.
Content has to be built around the means through which it is experienced.
Expecting a potential audience to engage with your out-of-date material and have the same reactions as they did in the past is nonsense. And it took a while for advertisers to realize this. For the longest time, it was believed that YouTube can only drive brand consideration and awareness, instead of immediate results.
However, slowly, advertisers began to learn that YouTube was the new television. Mastering YouTube would have been the same as mastering television back in the ’50s and 60s. Nevertheless, just as advertisers were beginning to feel comfortable in this new space, catastrophe struck.
In August 2016, YouTube experienced an advertiser mass exodus. YouTubers called this “the Adpocalypse.” It happened because prominent YouTubers were making risque content, the type of material which wouldn’t be permissible on TV, and eventually journalists began writing about it.
The public at large, which ultimately isn’t acquainted with the wildness of the wild web, was outraged and brands feared a backlash.
Long story short, advertisers decided to leave the site rather than having to deal with the fallout of indirectly funding potentially offensive content. Since then, there have been several “Adpocalypses,” wherein the end result is Google tightening the leash as to what content creators get monetized and promoted, with the goal of ensuring brand friendliness above all else.
The damage was done though, advertisers were spooked from the platform and they’ve been slow to pick the medium back up.
Fortunately for you though, this collective reticence makes it comparatively cheap to reach mass audiences on the site. And better still, the audiences on YouTube are very loudly signalling what they’re interested in by what content they engage with, and for how long.
How Google Does its Magic
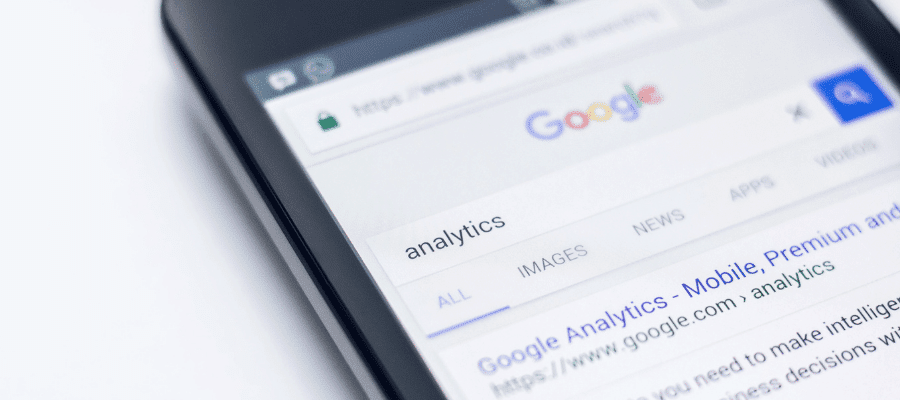
In order to understand YouTube Advertising, you need to understand Google itself and how it thinks. Because, after all, Google owns YouTube.
At the end of the day, all Google products are connected to the same black box. The only thing that changes between websites is the elements of that box that Google shows you. But don’t make the mistake of assuming that YouTube advertising is a completely different beast from regular Google advertising when it comes down to crunching the data.
Barring China, Google is the nexus of the world wide web. In one form or another, virtually every web user interacts with it in some capacity. And each of these people leaves their digital fingerprints all over the place.
Eventually, Google began to make note of these digital fingerprints to offer custom searches that best suit user preferences. For example, if I search “Danish” Google has to determine whether I mean the pastry or the ancestry. This used to be an impossible task when algorithms were dumb and simply searched for specific keywords, instead of trying to understand its users.
Even with users that search for the same content – say, news on a political candidate – there are preferences on the source. Some people have a strong preference for a particular newspaper, while others might want something more neutral like a Wikipedia entry.
In other words, for Google to best be able to show you what you’re likely to prefer, it has to understand your preferences in the first place. And oftentimes, people are not aware that they even have such biases!
So, Google began tracking this data in the hopes of understanding user preferences and service use. This generated trillions of data points that helped Google make educated guesses about their users.
For example, if you notice that certain types of behaviour happen between certain hours, you can make a reasonable assessment of what people are using the data for. If they’re searching for topics related to architecture between the times of 9-5 on weekdays, it’s not unreasonable to assume that they might be an architect.
This is an exponentially scalable data problem, as the variables within the models are self-referential (the fact that you might be able to collect some bit of data from some users is a data point in and of itself). Eventually, the whole matter became much too complicated for a human to realistically be able to understand – a team of top data analysts would fail to make sense of the data, even when given an eternity.
So, over the years, more and more decisions were passed on to algorithms. Which brings us to 2014, when Google bought an artificial intelligence company known as DeepMind.
DeepMind specializes in deep learning, wherein the AI operates via a feedback loop:
- First, it is given training data – millions of instances where a causal relationship is known “if there is X then Y happens” but not why that happens.
- Then, it starts building models with random and subtle variations to try to better explain the millions of instances.
- These models then start making predictions on live data, with which they try to predict the outcome thereof.
- After the outcome is known, it deletes the models with the least predictive power and it keeps trying to perfect itself by repeating the process, each time getting a tiny bit better.
In other words, the neural network functions like a black box, which nobody – not even its programmers – fully understand. It just takes trillions of self-referential data points, and through the countless generations of models, it creates an iterative system with evermore predictive power.
This technology allowed Google to better understand its users in order to make predictions on their needs and wants.
By understanding all its billions of users, Google is able to create demographic profiles for people. It’s worth stressing though that just because Google has decided you fit into the “Sports” Target Audience, it doesn’t mean you’re not also part of a host of other potential audiences like “Finance” or “Make-up.”
People are multidimensional and Google understands this.
Imagine, for example, that you’re a person who is interested in Sports, but because you have a date in the coming week, you’ve been trying recipes and watching cooking channels.
Now, if the system wasn’t able to understand the complexities of a person, it would only serve a bunch of cooking-related advertisements to you. But Google has also tagged you as someone interested in athleticism. So, even if you watch videos on how to bake a cake, you would still occasionally see sports-related advertisements.
When marketers think they can outsmart Google, they run into problems. Many marketers commonly assume that it’s best to build their potential product audiences from the ground up. They start tagging specific placements, keywords and whatnot, but as a single person without access to Google’s data-crunching capabilities, they might not be aware of nuanced correlations.
For instance, something about a certain profile makes it 10% more likely that a person will be interested in finance than the average public. Nobody knows why such a relationship happens at all, nothing about your profile screams that you might even like finance. But Google has noticed this relationship between people like you and an interest in finance.
Traditionally, if a statistician were to see this connection, they would likely say it’s a spurious relation and dismiss it. However, Google’s deep learning algorithms would start building models around that correlation. And if the models have predictive power, then it keeps those assumptions.
It’s incapable of understanding why it happens at all, it just knows that it consistently happens.
So, theoretically speaking, if someone were to ask Google to find people who might enjoy finance, it might include you in the audience, despite the fact that you have never shown an inclination before. A human being could not see this relationship between people with your profile and finance, let alone capitalize on it – the algorithm can.
As such, Custom Audiences artificially built by humans have the issue of drawing from a shallow pool, as you’re only using the things associated with the target audiences that you are aware of. In other words, you’re not using Google to its full capacity.
So, if you are interested in mass marketing, and not just looking for the archetypical ideal customer, then learning to use Google’s broader targeting, and In-Market audiences, is very useful.
The Different Ways Google Targets Audiences
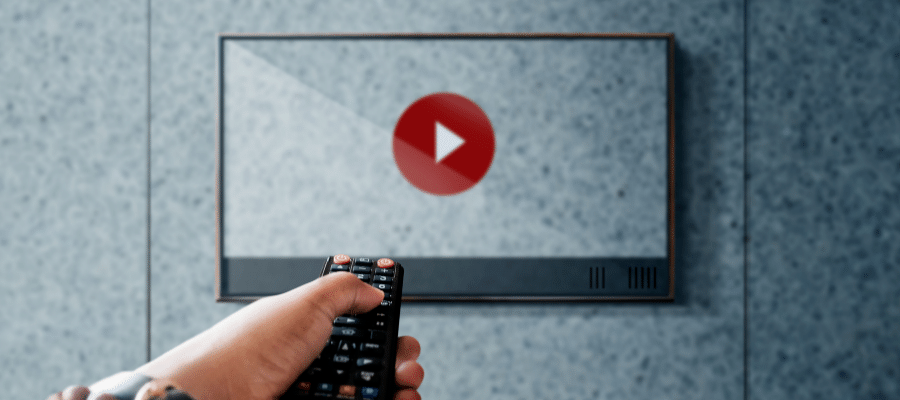
In an earlier section, we talked about the development of Google as an entity which can not only track the behaviour of all its users but also make sense of the trillions of data points and use the predictions it developed from interpreting the data.
Broadly speaking, there are two ways that Google finds audiences which we will be discussing throughout this next section.
Content-Based Targeting
This type of targeting essentially looks for specific instances of a phrase, or related terms (i.e., keywords), and matches it with tags that content creators add to their posts.
It primarily does so by scraping the metadata associated with a piece of content (information like genre, tags, author, etc.) and trying to match it with the keyword entered.
Before Google was able to understand user preferences, this used to be the primary means by which it searched for content to serve to its users. Content creators would helpfully provide titles, descriptions, genre, placement, etc. as metadata which helps the algorithm make the matches.
However, this approach eventually proved to be problematic. Content creators soon realized that there wasn’t anything stopping them from loading as many tags and keywords as they could to get more (or more profitable) eyeballs on their posts.
This is why “keyword stuffing” became prominent in the early days of Google. It got so bad that some content creators would add a list of keywords at the end of the articles but they would color the font the same as the background so that the list would be invisible to anyone but Google’s search spiders.
This naturally became an issue because you could make a dog grooming article look like a paper on nuclear physics to search algorithms if you stuff enough invisible keywords in it. Through this deception, webmasters could trick Google into paying higher rates for ad placements because advertisers assumed they were getting affluent audiences, rather than more mainstream ones.
Over time, this became less of an issue as Google got a firmer grasp of their audiences instead of relying on keywords and its search algorithms became more capable of detecting such malicious practices.
So, even if you are searching audiences based on keywords nowadays, it’s not as simple as Google matching the metadata that people put into the content, the keywords you put in and the audiences searching for this content. Instead, on the back end, there’s a complex assessment happening based on search signals and browsing history.
Pure content-based targeting is a bit of a relic of the past.
It exists in terminology but mainly as a way of signalling that your main means of targeting people are certain keywords, phrases, and places they visit – this is partially what Google refers to when you’re searching by “Similar Audiences”. However, Google still somewhat guides things behind the scenes, though less heavy-handed as it might otherwise do.
If you want a good example of what content-based searches or metadata searches used to look like, go to a government website, or do a library search. They likely haven’t updated their search algorithms in decades, and it’s a nightmare to try and find any relevant content.
On the other hand, if you want to leverage YoutTube Advertising to its full capacity, then you may want to consider Audience-Based Targeting.
Audience-Based Targeting
By now, you understand why Google saw the need to start creating in-depth profiles on its users. Once they had algorithms that could predict behaviours and needs with a reasonable degree of accuracy, they began segmenting the different audiences.
Now, instead of the blunt and manual approach of content-based targeting through which keywords were (often arbitrarily) mapped onto certain demographics, the data could speak for itself and create various audience types.
It’s similar in a way to how television used to create various audiences. But while that was much more general, subject to the whims of television executives, and often speculative in its assessments, Google makes conclusions based on an essentially limitless pool of information.
It’s a constantly evolving system which is analyzing behaviour patterns in real-time as they change. Furthermore, these audiences are broad, as they seek to find everyone who fits into a certain behavioural pattern.
As we’ve mentioned before, when Google decides to put you in one Audience segment, it doesn’t mean this is the only one Google has identified you as. People are multidimensional, they can have more than one interest, and one preoccupation.
There are four primary ways of grouping google audiences, (excluding the categorizations which don’t just rely on matching the behaviour of a known customer with that of a new lead, or using the metadata of the things they interact with):
- Detailed Demographics – Does exactly what it says on the tin (age, sex, location, etc).
- Affinity – This refers to people’s interests and tastes. For example, if you like burgers, enjoy smooth jazz, or are interested in a particular type of sport.
- In Market – People who are “in the market” for something. It can be as complicated as searching for a job or thinking about buying a new car. But the primary thing is that the algorithms have flagged a sudden change in your online behaviour which more often than not signals purchase intent.
- Life Events – This is a relatively recent addition, but it just goes to show to what extent Google is beginning to understand its users. As of 2018, you can target people that are undergoing a specific life event, like getting married, graduating or approaching retirement.
- Custom Audiences – Here you can mix and match all the previous ones together. For example, you could theoretically target a 25-year old male vegan, who likes burgers, wants to buy a new car and is getting divorced. This can get quite specific, so you need to be careful not to make the parameters so narrow that only a handful of people in the world match them (unless you are not selling a mass-market product and are only interested in a very specific type of potential client).
In other words, In-Market & Affinity audiences are as broad as they could be. Presently there are around 141 or so Affinity audiences, and 779 In-market audiences.
Google could naturally segment them into smaller, and smaller groups. But this goes against their interests. For starters, marketers could easily get overwhelmed. Imagine if instead of 150 affinity audiences there were 150,000!
Yes, they might be more specific, but to know that a certain category exists, your Google-fu would have to be extraordinarily good. Besides that, every single time they create a new category, the potential target audience shrinks that much more.
Let’s say you have an Audience called “Deadline Procrastinators,” and it includes me and basically everyone else I know. Now, if Google were to break this audience down into many smaller audiences it might initially sound like a good idea.
But let’s continue with that example, “Deadline Procrastinators” gets broken into a hundred other segments, among them “Lab Report Deadline Procrastinators” which logically would be a much smaller audience, and would hardly include anyone I know, as nobody has to file lab reports.
If I’m looking to sell a product to help procrastination, I don’t care what people are procrastinating. The hyper specificity of the audiences would actively harm me, as I’d have to find every single Audience that Google has created for procrastinators – and much the same applies to anyone selling a mass-market product.
On the other hand, if I am interested in selling a bespoke product, I have the Custom Audiences option.
So, Google has decided to go with the best of both worlds – audiences as broad as they could possibly be while having the option of customizing your needs into the system.
Conclusion
YouTube remains a relatively underappreciated space. Despite being a part of the Google ecosystem and being the second-most-visited site on the web, advertisers often shun it because they don’t know how to leverage it to its full capacity
This is useful for potential marketers because, compared to other media types, advertising on YouTube remains well worth the money. And unlike other more faceless advertising mediums, YouTube you can leverage parasocial relationships and engage with audiences more efficiently.
In addition to this, given that it’s under the Google umbrella, its advertising is managed by the Google Advertising suite, which carries with it immense benefits. You can target billions of users based on a wide range of factor, from the metadata of the content they interact with to their interests, life circumstances, demographic details, and much more.
As such, while professional marketers often focus on increasing their marketing efficiency by polishing their ad copy or reducing their funnel friction, they forget that only a certain percentage of their potential audience is likely to be interested in buying their product – it doesn’t matter how convincing the pitch is, some people, even if they’re the target audience are simply not interested in purchasing.
So, marketers obsess over their ideal audience and spend ever more money trying to pry them from their lethargy. But it is a bit of a losing battle.
When a lemon dries up, the smart thing is not to keep squeezing harder and harder to extract the last few drops but to simply get another lemon.
The fastest way to scale a mass-market product campaign is to figure out how to use the In-Market and Affinity Audiences, as through them you can essentially reach a limitless audience.
YouTube is the new TV, and video is the medium of the future. The marketers who decide to embrace this new reality will be the ones who will rise to prominence in years to come. Mastering YouTube now is like mastering TV back in the ’50s-’60s.
The world has changed, and we must change with it to keep up and thrive.
[…] Why YouTube Advertising is (Wrongfully) Overlooked […]